toxcast에서 0.805수준의 AUC 를 보여준 논문.
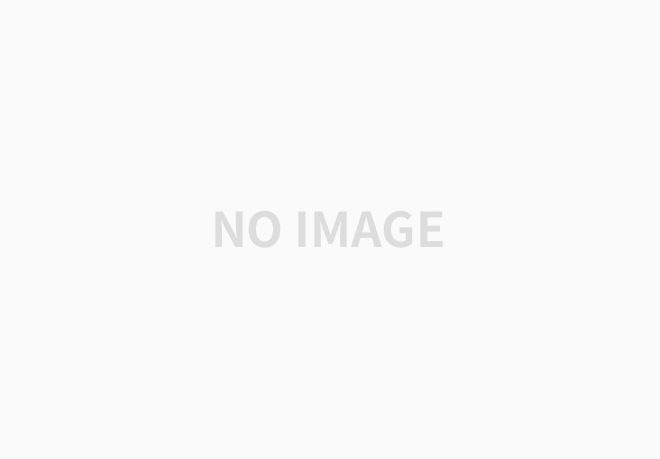
ABSTRACT:
Hunting for chemicals with favorable pharmacological, toxicological, and pharmacokinetic properties remains a formidable challenge for drug discovery. Deep learning provides us with powerful tools to build predictive
models that are appropriate for the rising amounts of data, but the gap between what these neural networks learn and what human beings can comprehend is growing. Moreover, this gap may induce distrust and restrict deep learning applications in practice. Here, we introduce a new graph neural network architecture called Attentive FP for molecular representation that uses a graph attention mechanism to learn from relevant drug discovery data sets. We demonstrate that Attentive FP achieves state-of-the art predictive performances on a variety of data sets and that what it learns is interpretable. The feature visualization for Attentive FP suggests that it automatically learns non local intramolecular interactions from specified tasks, which can help us gain chemical insights directly from data beyond human perception.
유리한 약리학 적, 독성 학적 및 약동학 적 특성을 가진 화학 물질을 찾는 것은 신약 개발에있어 여전히 어려운 과제입니다. 딥 러닝은 예측을 구축 할 수있는 강력한 도구를 제공합니다.
증가하는 데이터 양에 적합한 모델이지만 이러한 신경망이 학습하는 것과 인간이 이해할 수있는 것 사이의 격차가 커지고 있습니다. 더욱이 이러한 차이는 실제로 불신을 유발하고 딥 러닝 응용 프로그램을 제한 할 수 있습니다. 여기에서는 그래프주의 메커니즘을 사용하여 관련 약물 발견 데이터 세트에서 학습하는 분자 표현을위한 Attentive FP라는 새로운 그래프 신경망 아키텍처를 소개합니다. 우리는 Attentive FP가 다양한 데이터 세트에서 최첨단 예측 성능을 달성하고 학습 한 내용을 해석 할 수 있음을 보여줍니다. Attentive FP의 기능 시각화는 지정된 작업에서 비 로컬 분자 내 상호 작용을 자동으로 학습하여 인간의 인식을 넘어서는 데이터에서 직접 화학적 통찰력을 얻는 데 도움이 될 수 있음을 시사합니다.
'AI 독성예측' 카테고리의 다른 글
[AI독성예측] K-aiTox 연구회 (0) | 2021.05.02 |
---|---|
[논문분석] kGCN: a graph-based deep learningframework for chemical structures (0) | 2021.04.23 |
[AI독성예측] ToxCast란? (0) | 2021.04.10 |
[AI독성예측] 신약개발에서 딥 러닝의 부상 (0) | 2021.03.24 |
[AI독성예측] 컴퓨터를 이용한 신약개발 방법론(안전성평가연구소) (0) | 2021.03.23 |